Multi-Gene and Rule-Guided Classification of Influenza Viruses via Cellular Automata Genomic Image Transformation and Deep Learning
This research aims to establish a reliable classification framework for the influenza virus using genomic image transformation and deep learning. The system employs cellular automata to transform viral gene sequences into structured image formats, facilitating virus visualization, identification, and subtyping. This approach has the potential to generate a more accurate understanding of viral detection and evolution, particularly in the context of rapidly changing strains.
The project utilizes various techniques, including computational biology, bioinformatics, and artificial intelligence. It explores rule-based cellular automata image encoding, leverages cutting-edge neural network structures, and optimizes multi-task learning approaches. This comprehensive approach aims to enhance the accuracy and interpretability of the classifiers employed for viral genomes, thereby expanding our capacity to monitor pandemics and ultimately influencing virus genomics and public health intelligence.
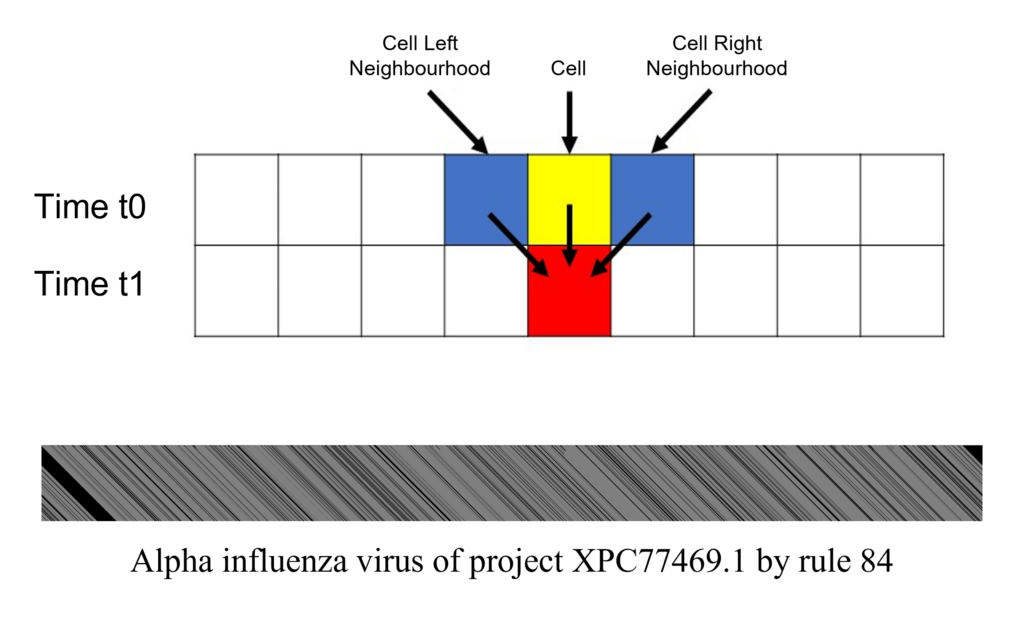