Automated Lumbar Intervertebral Disc Detection and Classification System Based on a Hybrid Deep Learning Framework
This study proposes a hybrid deep learning framework that integrates object detection and classification models to achieve a fully automated assessment of intervertebral disc health. The system first employs the YOLOv7 model to accurately locate intervertebral discs within DICOM images, extracting regions of interest (ROIs) while preserving anatomical features and eliminating irrelevant tissue interference. To enhance classification accuracy, data augmentation techniques such as rotation and contrast adjustment are applied. Additionally, transfer learning is utilized to fine-tune pre-trained models like ResNet18, addressing the challenge of limited medical image datasets.
To facilitate clinical application, an intuitive graphical user interface (GUI) is designed to seamlessly integrate the optimized model workflow. Users can upload medical images, and the system generates results within one second, automatically identifying intervertebral disc locations and classifying their health status. This automated approach enhances diagnostic efficiency and consistency, assisting clinicians in making faster and more accurate decisions.
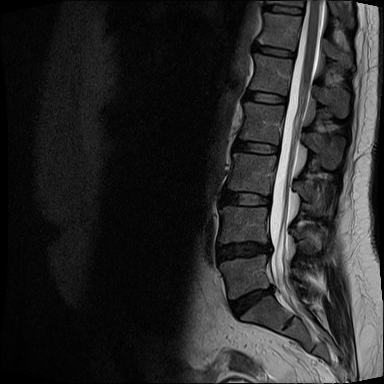