Automatic GBM and Brain Tumor Recognition and Classification in MRI Based on Semantic Segmentation and Deep Learning
Brain tumor detection and classification from MRI images are crucial tasks in medical imaging. This study focuses on utilizing semantic segmentation models and deep learning techniques to automate the segmentation and classification of various brain tumors, including Glioblastoma Multiforme (GBM), one of the most aggressive types. The research explores advanced segmentation models such as UNet, Swin-UNet, and YOLO-based architectures to accurately delineate tumor regions. Additionally, classification models including AlexNet, Vision Transformer, and Swin Transformer are employed to categorize different tumor types effectively.
The proposed approach leverages deep learning-based feature extraction and classification techniques to enhance accuracy and efficiency in brain tumor analysis. The dataset consists of annotated brain MRI images representing multiple tumor types, including GBM, which are preprocessed and augmented to improve model robustness. Various performance metrics, such as Dice similarity coefficient, Intersection over Union (IoU), accuracy, and F1-score, are used to evaluate the effectiveness of both segmentation and classification models.
By integrating semantic segmentation with deep learning-based classification, this research aims to provide a more accurate and automated solution for brain tumor diagnosis. The findings of this study can contribute to improving early detection and clinical decision-making, ultimately benefiting patients and medical professionals in the field of neuroimaging and oncology.
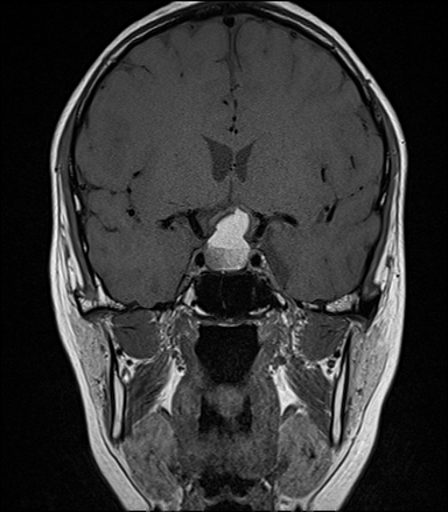