Deep Learning-Based Identification and Prediction of Comorbidity Risk Factors for Sepsis
Sepsis is a life-threatening systemic inflammatory response syndrome, often triggered by infection, which can rapidly progress to multiple organ failure and death. Clinical observations have shown that many patients develop sepsis in the presence of one or more chronic comorbidities, such as diabetes, chronic kidney disease, or cancer. These comorbidities may significantly influence both the risk and outcomes of sepsis.
This study proposes a deep learning-based framework for the identification of comorbidity risk factors and the prediction of sepsis onset, utilizing the MIMIC-IV clinical database. By integrating diagnostic codes and clinical data—such as vital signs and laboratory results—the proposed framework leverages various machine learning and deep learning models to analyze the contribution of different comorbidities to sepsis development. The model incorporates explainability techniques such as SHAP to interpret predictive factors and uncover high-risk disease patterns, thereby enhancing the accuracy of early sepsis risk prediction.
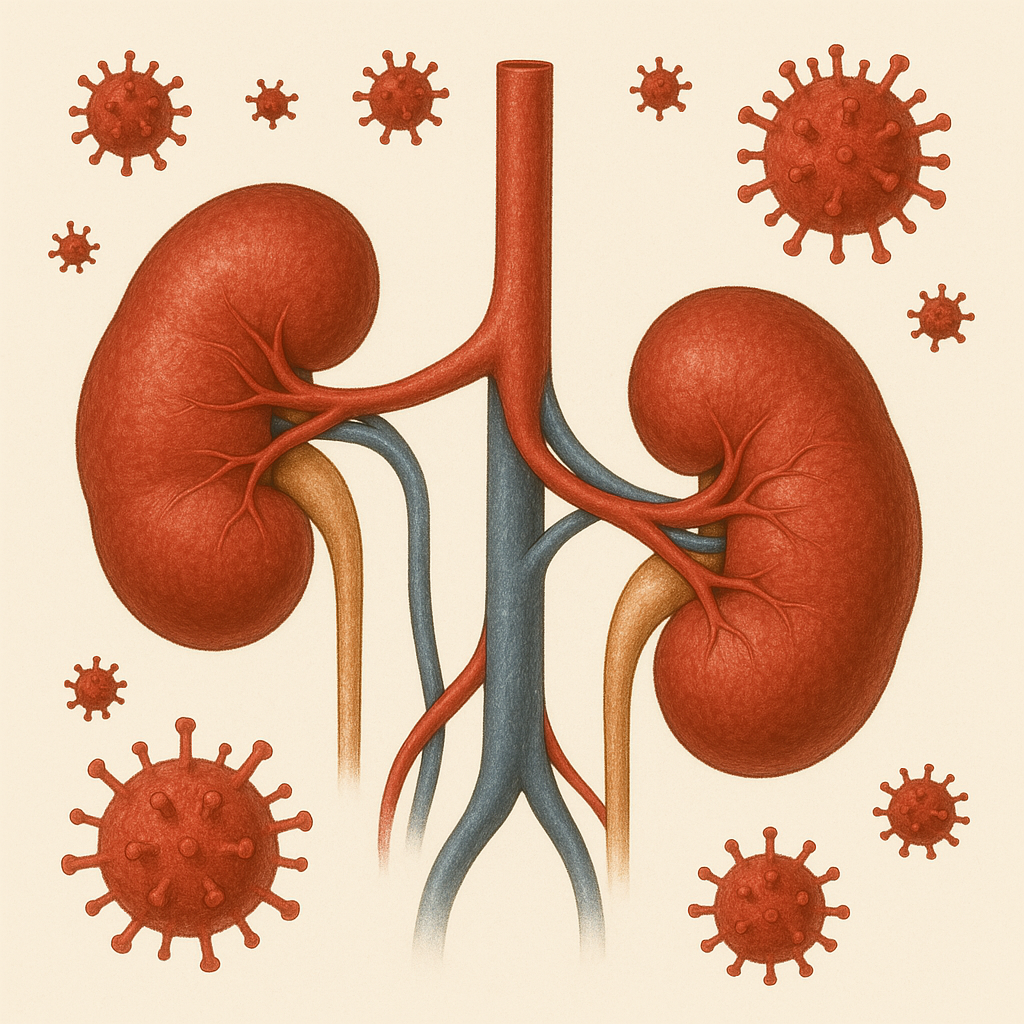