Multi-Omics Data Integration and Classification for Alzheimer’s Disease Based on Multi-Layer Attention Graph Learning
Alzheimer’s disease is a common neurodegenerative disorder and the leading cause of dementia in the elderly, characterized by memory loss, language difficulties, and cognitive decline. Its causes involve abnormal brain protein accumulation, genetics, and aging. While there is no cure, early detection can slow progression and improve quality of life.
This study proposes a multi-omics data integration and classification framework based on multi-layer attention graph learning. By integrating heterogeneous omics data such as gene expression, DNA methylation, and proteomics, the model employs graph representations and attention mechanisms to effectively capture potential relationships among data types, thereby enhancing the accuracy of Alzheimer’s disease classification. This approach contributes to the discovery of potential biomarkers and facilitates early diagnosis and understanding of disease mechanisms.
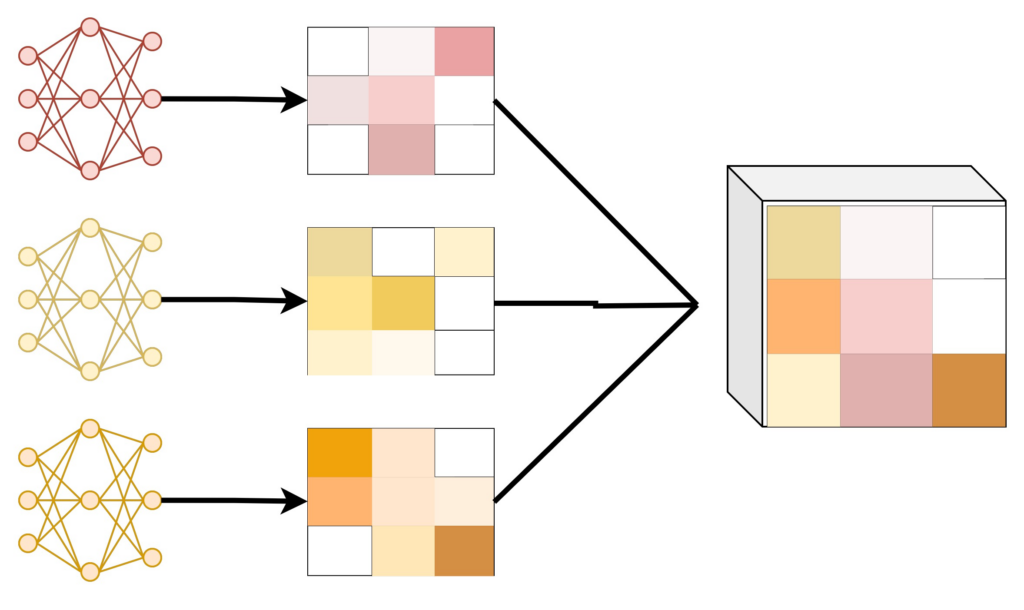